Accessing and understanding company policies has always been a challenging task for employees. According to a Gartner survey, 47% of digital workers struggle to find the information needed to effectively perform their jobs. Finding the right documents on intranet sites and sifting through pages of information to locate specific answers can be a tedious process. This becomes even more complicated for larger companies operating in multiple countries with localised policies.
When my start-up was acquired by a large multi-national and I became 1 of 9,000 employees, I was reminded of the frustrations involved in finding company information. The company had grown by acquisition, so not only was there country-specific policies but differing versions of handbooks and documents within countries. Moreover, Finance, HR and Operations provided their own separate intranet sites. Even small firms with a few hundred employees face similar challenges.
However, the introduction of Gen AI and Large Language Models (LLMs) is set to transform this experience, providing a more efficient way for employees to access vital information.
The Traditional Approach
Traditionally, employees needing to consult their HR handbook, expenses policies, or other company documents faced a laborious journey. They would first need to navigate through complex intranet sites to locate the correct document. Once found, the task of manually searching through dense text begins. This method is not only time-consuming but also increases the risk of misinterpretation, especially when dealing with localised policies in different regions.
Introducing LLMs
Large Language Models, such as ChatGPT, Microsoft Copilot, or Google Gemini represent a significant advancement in how information is accessed and processed. These models can understand and generate human-like text, making it possible for them to interact with users in natural language. When integrated with a company’s document store using a technique known as Retrieval Augmented Generation (RAG), LLMs can transform how employees query and retrieve information.
The RAG technique enhances the capabilities of Large Language Models by combining them with a retrieval system. This approach allows the model to access a vast store of documents or data in real-time, retrieving the most relevant information to generate more accurate and contextually appropriate responses. By leveraging both generative and retrieval-based methods, RAG ensures that the answers provided are not only generated based on learned patterns but are also grounded in specific, up-to-date information from the document store. This significantly improves the accuracy and reliability of the information returned by the LLM.
Transforming Information Access
LLMs improve the employee experience by allowing natural language queries via a simple interface. Employees can ask questions like, "What is my holiday allowance?" and receive instant, accurate answers. This eliminates the need to search through multiple documents or sites manually. The consistency and accuracy of LLMs, particularly when made to reference their sources, ensure that employees receive the correct information every time, reducing the risk of misunderstandings.
Furthermore, LLMs can handle updates to policies seamlessly. Once a policy is updated in the document store, the LLM can immediately reflect these changes in its responses, ensuring that employees always have access to the most current information.
Real-world Applications
Consider a multinational company that has implemented LLMs to improve knowledge worker productivity. Employees across different regions can now access localised policies by simply asking the LLM, regardless of the country they are in, removing the need to provide a local intranet site or go hunting for the relevant policy. This not only saves time but also improves overall efficiency and employee satisfaction.
Scalability is another major advantage. LLMs can be tailored to fit the specific needs of any organisation, from mid-sized enterprises to large corporations. Customisation allows for integrating specific company jargon and policies, making the system more intuitive for employees.
Solutions are readily accessible and easy to implement, particularly for companies using Microsoft 365 with SharePoint or Google's Workspace. Copilot from Microsoft and Gemini from Google provide natural language searching for documents on the file store with minimal configuration required. OpenAI's ChatGPT for Enterprise can also be attached to enterprise data to perform the same function.
Addressing Concerns
Implementing LLMs does come with concerns, particularly regarding data security and privacy. Companies must ensure that the integration of LLMs adheres to strict data protection protocols. Using encryption and secure access controls can mitigate these risks, ensuring that sensitive information remains protected.
Services like Microsoft M365 Copilot, Google Workspace and ChatGPT for Enterprise make this easy to configure and deploy. Organisations could also implement their own custom on-premise or cloud hosted LLM connected to a datastore. While this approach offers greater security and privacy, it is more difficult to implement and has a higher maintenance overhead.
Cost and deployment are also considerations. While there is an initial investment involved, the return on investment can be significant. The reduction in time spent searching for information and the increase in productivity will most certainly offset the costs in a short timeframe. Additionally, many vendors offer support to ease the integration process with existing systems.
Are The Days of the Intranet Numbered?
Large Language Models are set to transform how employees access and understand company policies and other information. By providing a single easy-to-use interface that delivers instant, accurate answers to natural language queries, LLMs make the process of retrieving information more efficient and less error-prone.
In the near future, those capabilities will be further enhanced through the use of GPTs or Gems that are customised for a company's specific needs. And the forthcoming agents like OpenAI's GPT-4o assistant and Google's Project Astra, will provide a rich experience for employees. For example, a single voice command "I need to book a week off in July. Can you remind me how many days holiday I get each year, how many I have left and book off the week of 12th July" will trigger the agent to look up information from multiple sources, book your holiday into the system and block out your calendar.
As companies continue to adopt these technologies, the future of workplace information access looks promising and the frustrating experience of trawling corporate intranets to find information a thing of the past.
Organisations interested in enhancing their employee experience should consider exploring the benefits of LLMs and how they can be integrated into their existing systems. Contact Pivotal Edge AI today to learn how we can help you deploy LLMs for efficient information retrieval in your organisation.
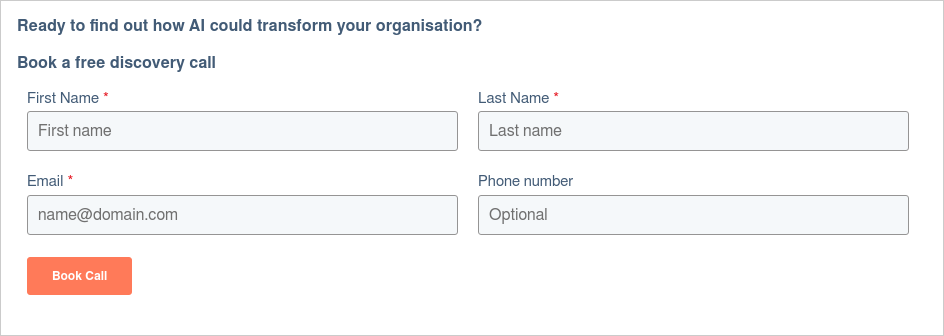
Tags:
BlogThu, May 16, 2024